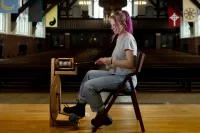
Microsoft’s Susan Dumais ’75 is a big reason why, computer-wise, you find what you seek
The next time you get a good answer from a web search, you can thank Susan Dumais ’75.
Beginning with landmark research in the 1980s, Dumais has made fundamental contributions to how we find, use and make sense of information on the Internet and in our computers.
For her achievements, Dumais was recently named the 2014-15 Athena Lecturer by the Association for Computing Machinery’s Council on Women in Computing.
“Her sustained contributions have shaped the thinking and direction of human-computer interaction and information retrieval,” said Mary Jane Irwin, who heads the Athena Lecture awards committee.
The Athena award celebrates female researchers who have made fundamental contributions to computer science.
The author of more than 200 articles on information science, human-computer interaction and cognitive science, Dumais is a Distinguished Scientist and deputy managing director of the Microsoft Research Lab in Redmond, Wash.
A native of Lewiston who grew up on Nichols Street near campus, Dumais was a magna cum laude mathematics and psychology major at Bates.
As a student, she did research with Drake Bradley, the Charles A. Dana Professor Emeritus of Psychology, co-authoring a research article on subjective contours that appeared in the journal Nature.
Being published as a student? “Pretty awesome,” she recalls. The pair also explored ways to use computer software to improve the teaching of statistics.
“She was one of the best students I ever had,” says Bradley, a comment echoed by Robert Moyer, professor emeritus of psychology, who says that Dumais “was one of the very best researchers I ever worked with — student or otherwise.”
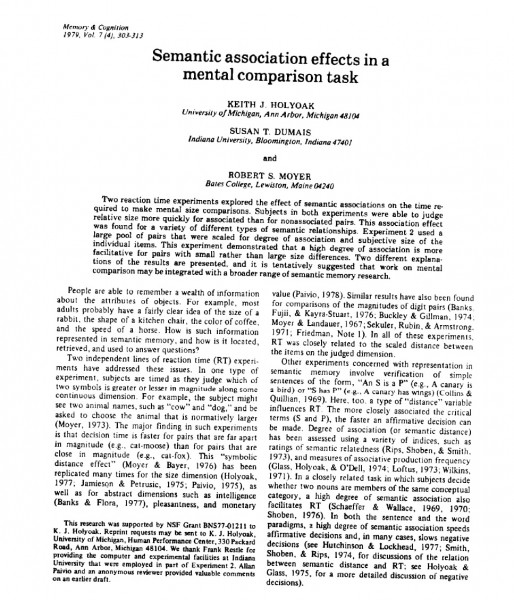
Research with Bates professors in the 1970s, including this article with Robert Moyer, presaged Dumais’ work to shape the direction of human-computer interaction and information retrieval.
Dumais headed to Indiana University for doctoral work, but didn’t leave Bates behind.
In 1979, she teamed with Moyer and a University of Michigan researcher to publish the article “Semantic association effects in a mental comparison task,” in the journal Memory & Cognition.
The researchers measured how long it took subjects to say which item in a pair was larger, such as flea or dog, needle or boat, penguin or iceberg.
Subjects were able to judge the size of semantically related terms (such as dog and flea) more quickly than unrelated terms (needle and boat).
To be sure, the researchers’ conclusions went deeper than that, but the thrust of Dumais’ work — modeling how human memory works — was a leaping-off point for a career exploring how humans can better communicate with their machines.
(Fun Bates connection: At Indiana, Dumais met a “fantastic teacher doing research in biopsychology” who joined the Bates faculty the next year. That professor was John Kelsey, who retired in 2012 after a great career at Bates.)
Ph.D. in hand from Indiana, Dumais was on her way. After looking at how humans retrieve information from our own memories, she began looking at how we retrieve information from external sources, such as computers.
In the 1980s, Dumais was researcher at Bell Labs when she co-authored a paper exploring “the vocabulary problem,” the fact that computer users always had to key in the exact, correct word to get what they wanted from their machine.
Reading like a manifesto for the information age, the paper explained the problem:
Many functions of most large [computer] systems depend on users typing in the right words. New or intermittent users often use the wrong words and fail to get the actions or information they want. This is the vocabulary problem. It is a troublesome impediment….In what follows we report evidence on the extent of the vocabulary problem and propose both a diagnosis and a cure. The fundamental observation is that people use a surprisingly great variety of words to refer to the same thing.
From that observation came a solution, Latent Semantic Indexing, published three years later in the journal of the American Society for Information Science with Dumais as a co-author.
Today, in terms of how we find stuff on the Internet, whether cute kittens or Red Sox highlights, LSI acts as an indexing and retrieval method. It helps our search experience by deciding if words that share similar contexts also share a similar meaning, and thus should be elevated as a search result.
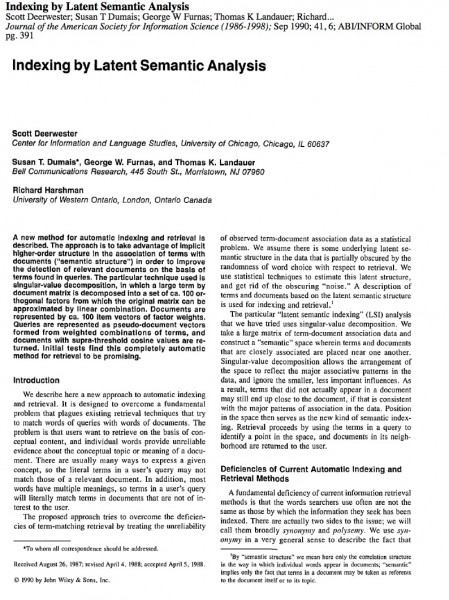
Dumais was a co-author of the seminal 1990 paper that described Latent Semantic Indexing, a revolutionary way to index and retrieve information.
“For example,” Dumais says, “LSI would find that the words ‘physician’ and ‘doctor’ are similar not because they co-occur together but because they occur with many of the same words, such as ‘nurse,’ ‘patient,’ or ‘hospital.'”
Essentially, she adds, “LSI induces the similarity among words by looking at the company they keep.”
Dumais’ most recent research at Microsoft analyzes how web content changes over time, how people revisit web pages, and how searching can be improved by modeling the context of the search.
By context, she means “who is asking, what task they are trying to accomplish, and what documents are of interest.”
That means you get your own search results. “So if I, Susan Dumais, search for SIGIR, I probably mean the ‘Special Interest Group on Information Retrieval’ and not the ‘Special Inspector General for Iraq Reconstruction.'”
In a good sense, Dumais sees no end to the work ahead.
The ability to search for information, she says, will support an “ever-increasing range of information, services and communications.”